What AI to use? The AI Showdown: Generative vs. Traditional
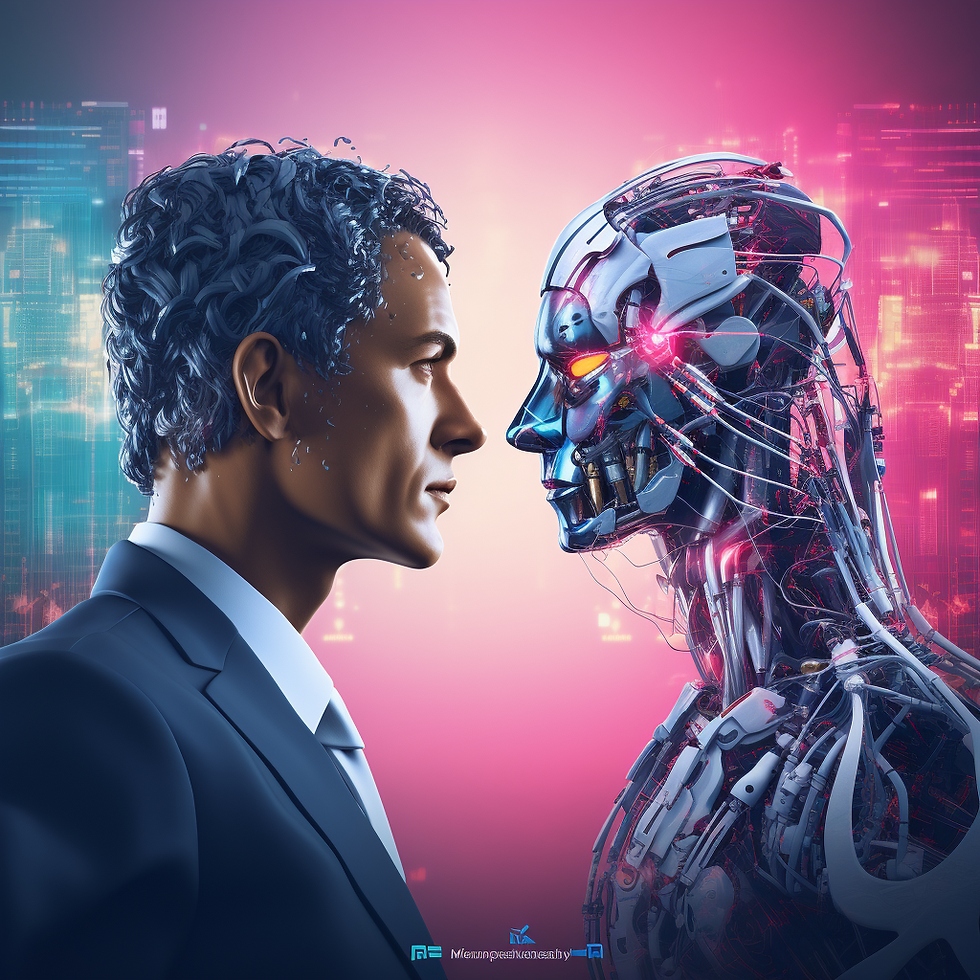
Artificial intelligence (AI) is transforming industries and redefining how humans interact with technology. Within the AI landscape, two distinct standards have emerged: generative AI and traditional AI. While both offer immense potential, they have fundamental differences in their approach and applications. This article provides an in-depth comparison between these two AI models.
What is Generative AI?
Generative AI represents a paradigm shift - AI that can generate new content and demonstrate open-ended creativity. Building on unsupervised and reinforcement learning, generative models do not need explicit human supervision or labelling. They learn undiscovered patterns and structures within unlabeled training data. Generative AI refers to AI systems capable of creating new, original data that is similar to data from the real world. It leverages unsupervised learning techniques like deep learning to discover patterns in training data and generate new examples that plausibly represent the original data distribution.
Unlike traditional AI, generative AI does not rely on hard-coded rules or human supervision. Instead, it autonomously learns representations of data and uses that knowledge to produce new samples. Some examples of generative AI include:
Generative adversarial networks (GANs): GANs can generate photorealistic fake images of people, objects, and landscapes. Nvidia recently unveiled an AI system called GauGAN that can turn simple sketches into photorealistic landscapes.
Autoregressive models: Models like GPT-3 use autoregressive algorithms to generate logical, human-like text given a prompt. GPT-3 has produced articles, poems, code, and conversational text.
Deepfakes: By swapping faces in videos, deepfakes leverage generative AI to produce compelling fake videos. While raising ethical concerns, this demonstrates the technology's creative potential.
AI art: Models like DeepDream use neural networks to generate unique, abstract artworks through pattern recognition and image manipulation.
What is Traditional AI?
While Traditional AI has seen waves of hype and promise since its origins in the 1950s, practical success was limited for decades. This changed with the machine learning revolution of the 2000s. By learning from examples rather than following rigid programs, machine learning allowed AI systems to tackle complex real-world tasks like computer vision and speech recognition. Traditional AI (It is often called Predictive AI), on the other hand, shines in analyzing existing data to forecast future events, thereby enabling informed decision-making, cost reduction, and risk mitigation. Traditional AI algorithms are explicitly programmed by humans to perform well-defined activities. Some examples include:
Credit Risk Analysis in Banking: A bank uses Traditional AI to analyze applicant data and automatically assign credit risk scores to mortgage loan applications, expediting approval decisions and reducing default rates.
Food Aggregator: A food aggregator uses Traditional AI to forecast order volumes by analyzing historical orders, weather data, and local events to optimize driver scheduling and food inventory.
Logistics/Supply Chain: A logistics company uses Traditional AI to estimate future shipment delays by analyzing historical traffic patterns, weather data, and supply chain disruptions to proactively alert customers.
Industrial robots: Robots in manufacturing follow predefined programs to repeatedly perform tasks like assembly or welding with speed and precision.
Virtual assistants: Intelligent assistants use NLP and predetermined rules to understand natural language queries but do not have true language understanding capabilities.
Self-driving cars: Automated vehicles rely on computer vision and deep learning models to perceive and navigate their environment but cannot transfer that skill to other complex domains.
Key Differences
Here are some major differences between the two AI approaches:
Learning style: Traditional AI uses supervised learning, requiring humans to label training data. Generative AI can leverage both supervised and unsupervised techniques. It excels at unsupervised learning to find hidden patterns.
Goals: Traditional AI aims to optimize performance on specialized tasks. Generative AI focuses on creating novel, original content reflecting patterns in data.
Adaptability: Since traditional AI depends on rules and human oversight, adapting it to new domains is difficult. Generative models are more flexible and creative.
Explainability: The rule-based nature of traditional AI makes its decisions interpretable. The complexity of generative AI models limits explainability.
Maturity: Having been researched for decades, traditional AI is more mature and well-tested currently. Generative AI remains an emerging field with rapid innovations.
Which is Better?
There is no blanket answer to which approach is "better" — each has suitable applications based on specific requirements. Traditional AI surpasses human capabilities in narrow domains. Generative AI holds promise for creative and adaptive solutions but can be unpredictable or biased based on its training data.
For the foreseeable future, both generative and traditional techniques will continue to co-evolve and be combined in innovative ways. Connect with our AI Experts to explore more insight into where your business will benefit from AI and how.
Opmerkingen